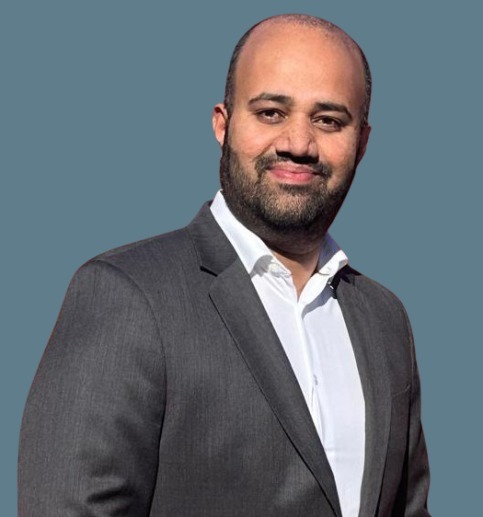
Mr. Sunilkumar Patel
Modern Clinical Statistical Analysis Modeling Techniques
The landscape of clinical research has rapidly evolved with advancements in statistical methodologies, enabling more robust, efficient, and nuanced analyses. This paper explores modern statistical modeling techniques applied within clinical studies, focusing on their role in enhancing precision, reliability, and interpretability of results. Key methods discussed include generalized linear models (GLMs), survival analysis models such as Cox proportional hazards, and more recent approaches like mixed-effects models and machine learning algorithms. We review their applications in clinical trial data, observational studies, and personalized medicine, emphasizing the strengths and limitations inherent to each technique. Additionally, the use of Bayesian statistics is highlighted for its growing importance in adaptive clinical trials and decision-making processes. The challenges of high-dimensional data, handling missing data, and ensuring model validation are addressed, alongside the integration of real-world data to augment traditional study designs. Through case studies and simulations, this paper illustrates the impact of these models in yielding actionable insights and supporting evidence-based Health care solutions. Finally, we outline future directions, including the integration of artificial intelligence with statistical models and the need for cross-disciplinary collaboration to advance clinical analytics.