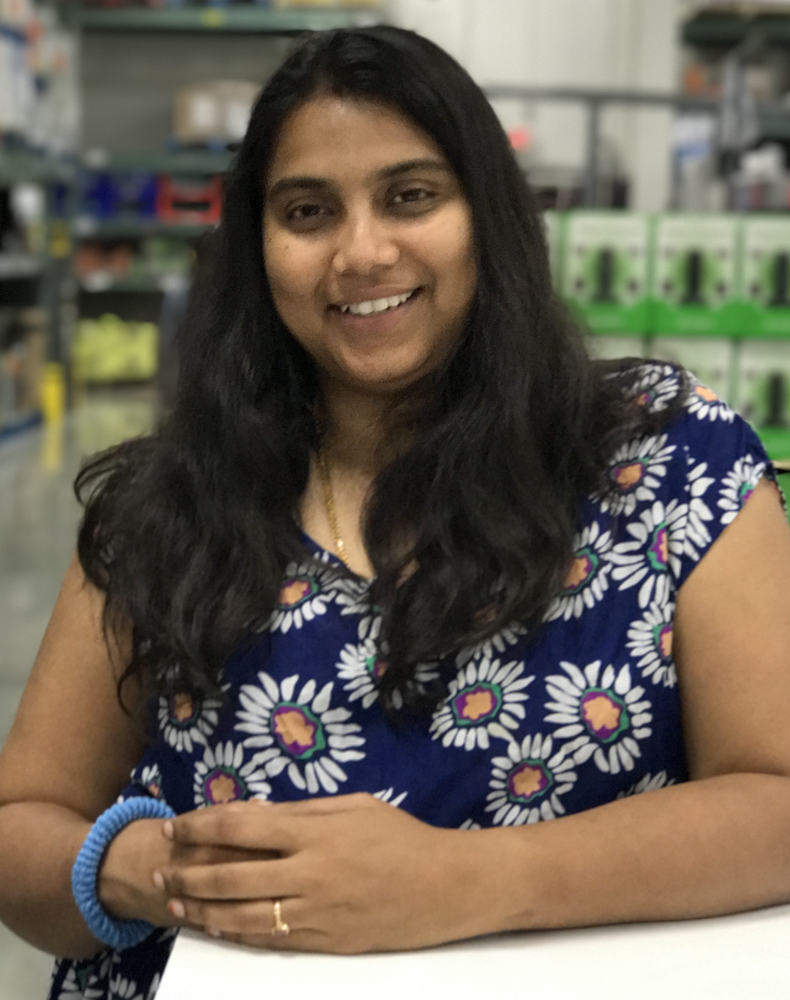
Ms. Naga Harini Kodey
Data Quality for Machine Learning in the AdTech Space
Abstract:
In the AdTech industry, machine learning is at the heart of real-time decision-making—from optimizing ad placements to predicting user behavior. But no matter how advanced the models are, their effectiveness hinges on one critical factor: the quality of the underlying data. As a Principal QA Engineer, I’ve seen firsthand how poor data quality can lead to inaccurate targeting, wasted ad spend, and misleading insights. This session will focus on the QA techniques and testing strategies required to uphold data quality across the full lifecycle of ML pipelines in AdTech. We’ll explore how to systematically test raw event data, validate complex feature engineering logic, and detect silent data failures that often go unnoticed until they impact model performance. Emphasis will be placed on handling high-volume, high-velocity data, preventing data drift, and ensuring that training and production environments remain consistent and reliable. Attendees will get key take-aways of the actionable frameworks for implementing end-to-end data validation, integrating automated checks into CI/CD pipelines, and working cross-functionally with ML and data teams to build trustworthy, production-ready models. If you’re working on advertising platforms, personalization engines, or predictive campaign analytics, this talk will offer practical tools to strengthen the foundation that machine learning relies on: clean, high-quality data.